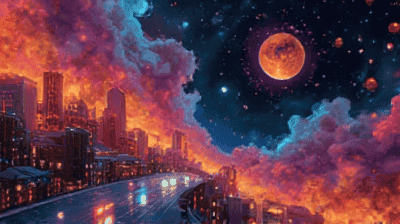
In recent years, artificial intelligence has made significant strides in creative fields, particularly in generating art. One of the most exciting developments in this area is the use of diffusion models to create stunning images from textual descriptions. This innovative approach allows for the synthesis of visuals that not only reflect the content of the provided text but also demonstrate artistic flair and complexity.
The Rise of AI in the Creative Arts
The advent of AI has brought about transformations in various creative domains, including music, literature, and visual arts. AI systems have demonstrated the ability to produce content that rivals human creativity, leading to intriguing discussions about the nature of creativity itself. Among these advancements, diffusion models stand out as a groundbreaking technique in generating images from text prompts.
Understanding Diffusion Models
Diffusion models are a class of generative models that learn to create data by gradually transforming noise into structured outputs. They operate on the principle of diffusion processes, where data is slowly perturbed by random noise, and then the model learns to reverse this process, ultimately generating new data that resembles the training data distribution.
The Process of Diffusion
Forward Process: In the first stage, the diffusion process adds noise to the training data over several time steps. This gradual introduction of noise transforms the original image into a pure noise representation. Mathematically, this can be represented as a Markov chain, where each step increasingly obscures the original data.
Reverse Process: In the second stage, the model is trained to reverse the diffusion process. It learns to denoise the data step by step, starting from random noise and aiming to reconstruct the original image. This is achieved through a series of neural network applications that predict the denoised version at each stage.
Sampling: Once trained, the model can generate new images by sampling from the noise distribution and applying the learned reverse diffusion process. The result is a new image that embodies the characteristics of the training data and can be guided by textual prompts.
Key Components of Diffusion Models
To understand diffusion models in depth, it is essential to explore their key components:
Neural Networks: At the core of diffusion models are deep neural networks, particularly convolutional networks, which are adept at learning complex patterns within data. These networks perform the denoising tasks and guide the generation of images.
Latent Space: Diffusion models operate in a latent space, a high-dimensional representation where the main features of data are captured efficiently. Navigating this space allows for the generation of diverse and coherent images.
Training Dataset: The quality and diversity of the training dataset significantly influence the output of diffusion models. A well-curated dataset will result in a wider array of visual styles and content in the generated images. Common datasets used for training include large collections of images with corresponding textual descriptions.
The Workflow of Generating Images from Text
Generating images from text using diffusion models involves several key steps:
Text Encoding: The first step is to convert the input text into a numerical representation that the model can process. This is typically accomplished using pre-trained language models like BERT or CLIP, which transform the textual input into an embedding in a meaningful vector space.
Conditioning the Model: The encoded text acts as a condition for the diffusion process. During training, the model learns to associate specific text embeddings with particular visual features.
Diffusion Sampling: After conditioning the model with the text representation, the diffusion process is initiated. The model begins with random noise and incrementally refines it based on the learned associations to generate an image that aligns with the input text.
Post-processing: The generated image may undergo post-processing to enhance its quality or artistic attributes. This step can include techniques such as upsampling, color adjustments, and style transfers.
Applications of AI Generated Art
The utilization of diffusion models for generating images from text has opened new avenues in various fields:
Art and Design: Artists and designers are using AI-generated art as a source of inspiration or as a collaborative tool in their creative processes. These models can produce unique visuals that spark new ideas and directions in artistic projects.
Advertising and Marketing: Businesses are employing AI-generated images for advertising campaigns, marketing materials, and social media content. The ability to generate customized visuals swiftly can significantly enhance engagement and branding efforts.
Game Development: In the gaming industry, diffusion models can be used to create assets dynamically, ranging from character designs to landscapes based on narrative prompts, allowing for more immersive and interactive experiences.
Virtual and Augmented Reality: Diffusion models can contribute to generated environments in virtual and augmented reality applications, providing users with rich and diverse visual experiences that enhance interactivity.
The Community and Cultural Impact
The advent of AI-generated art, particularly through diffusion models, is not just a technological advancement; it also engages with cultural and philosophical questions about creativity, authorship, and the role of machines in the artistic process.
Changing Definitions of Art: As AI-generated images gain recognition in galleries and exhibitions, the definition of art is evolving. The question arises: Can machines create art, or are they merely tools for human expression?
Collaboration Between Humans and AI: Many artists view AI as a collaborator rather than a replacement. This relationship invites discussions about the merging of human intuition and machine precision, creating hybrid forms of creativity.
Access to Art Creation: AI-generated art democratizes art creation, making it accessible to individuals who may not have traditional artistic skills. This expansion of creativity could lead to a broader appreciation for diverse forms of expression.
Challenges and Ethical Considerations
While diffusion models and AI-generated art present exciting possibilities, they also raise important challenges and ethical considerations:
Authenticity and Ownership: As AI-generated images permeate the art world, issues of authenticity and ownership arise. Questions about who owns the rights to AI-generated art—the artist, the programmer, or the machine—persist.
Bias and Representation: The training datasets used to develop diffusion models can carry biases that may result in the generation of images lacking diversity or representation. It is crucial to address these biases to create equitable AI systems.
Potential for Misuse: AI-generated art can be misused for malicious purposes, including the creation of deepfakes or misleading imagery. This potential misuse raises concerns about the ethical boundaries of generating art.
Impact on Traditional Artists: The integration of AI in the art world may challenge traditional artists' roles and livelihood. Balancing technological integration with support for human artistry will be vital in addressing these concerns.
The Future of AI Generated Art
As technology progresses, the future of AI-generated art through diffusion models appears promising. We can anticipate several trends and developments:
Enhanced Algorithms: Future advancements will likely lead to more refined and sophisticated diffusion models capable of generating higher quality images while requiring fewer resources.
Real-time Generation: We may see the development of real-time generation capabilities, allowing users to input text prompts and receive instant visual outputs, enriching interactive experiences.
Improved Personalization: As AI systems learn user preferences, they may become better at generating personalized art tailored to individual tastes and aesthetics, fostering deeper engagement.
Integration of Multimodal Systems: The combination of text, audio, and visuals in AI systems might create immersive storytelling experiences where users interact with rich, generative environments.
Collaborative Platforms: We could see the emergence of platforms that encourage collaboration between artists and AI, enabling a fusion of human creativity with machine-generated content.
Conclusion
The evolution of AI in generating art, particularly through diffusion models, signifies a crucial intersection of technology and creativity. As these models learn to translate text into stunning images, they challenge our understanding of art, authorship, and the role of machines in creative processes. While AI-generated art offers exciting opportunities for expression and innovation, it also prompts important discussions about ethical considerations and the future of human artistry.
As we move forward into this new era of creativity, embracing collaboration between humans and AI will be key to unlocking the full potential of artificial intelligence in the arts. The journey of AI-generated art is just beginning, and its impact on culture and creativity has the potential to shape our world in profound ways.